Computational Neurology
Computationale Neurologie
Klinik und Poliklinik für Neurologie
Dr. Xenia Kobeleva
In order to answer clinically relevant questions, computational neurology combines neuroimaging and clinical data. Computational neurology combines a data-based approach and a modelling-based approach; this integration not only allows for contributions to better understand neurological diseases, but also to improve diagnostics and therapies in neurology. The data-based approach assesses neurodegenerative diseases by using machine-learning techniques in order to associate clinical phenotypes with characteristics of brain structure and function.
The modelling-based approach builds on these insights. Using mathematical concepts, this approach creates mechanistic and interpretable relationships between clinical data and brain activity, which is beyond a pure descriptive value. These kinds of models can be used to test potential treatments in a virtual setting. Therefore, computational neurology contributes to a deeper understanding of neurological diseases and to the development of new diagnostics and therapies.
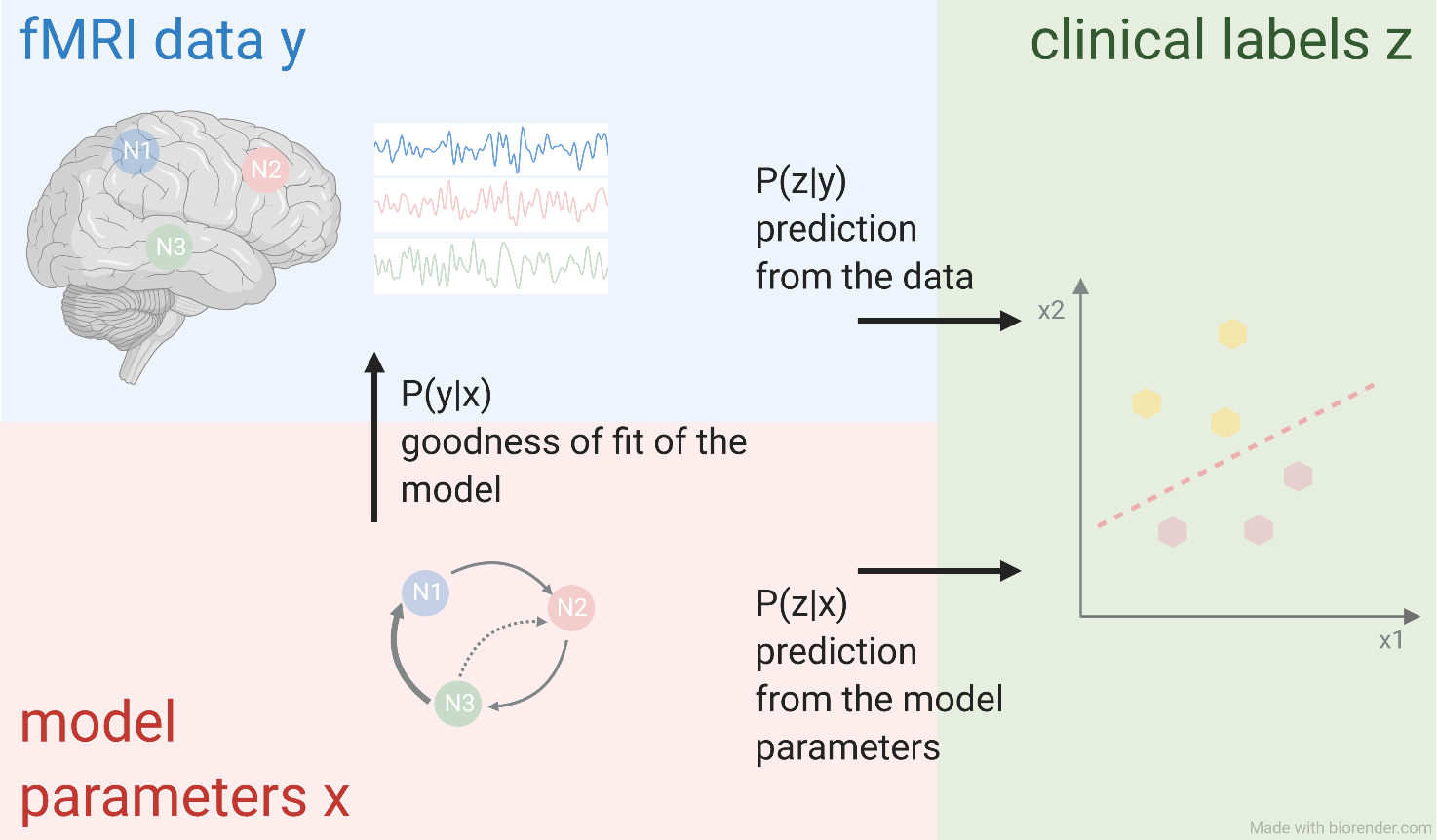
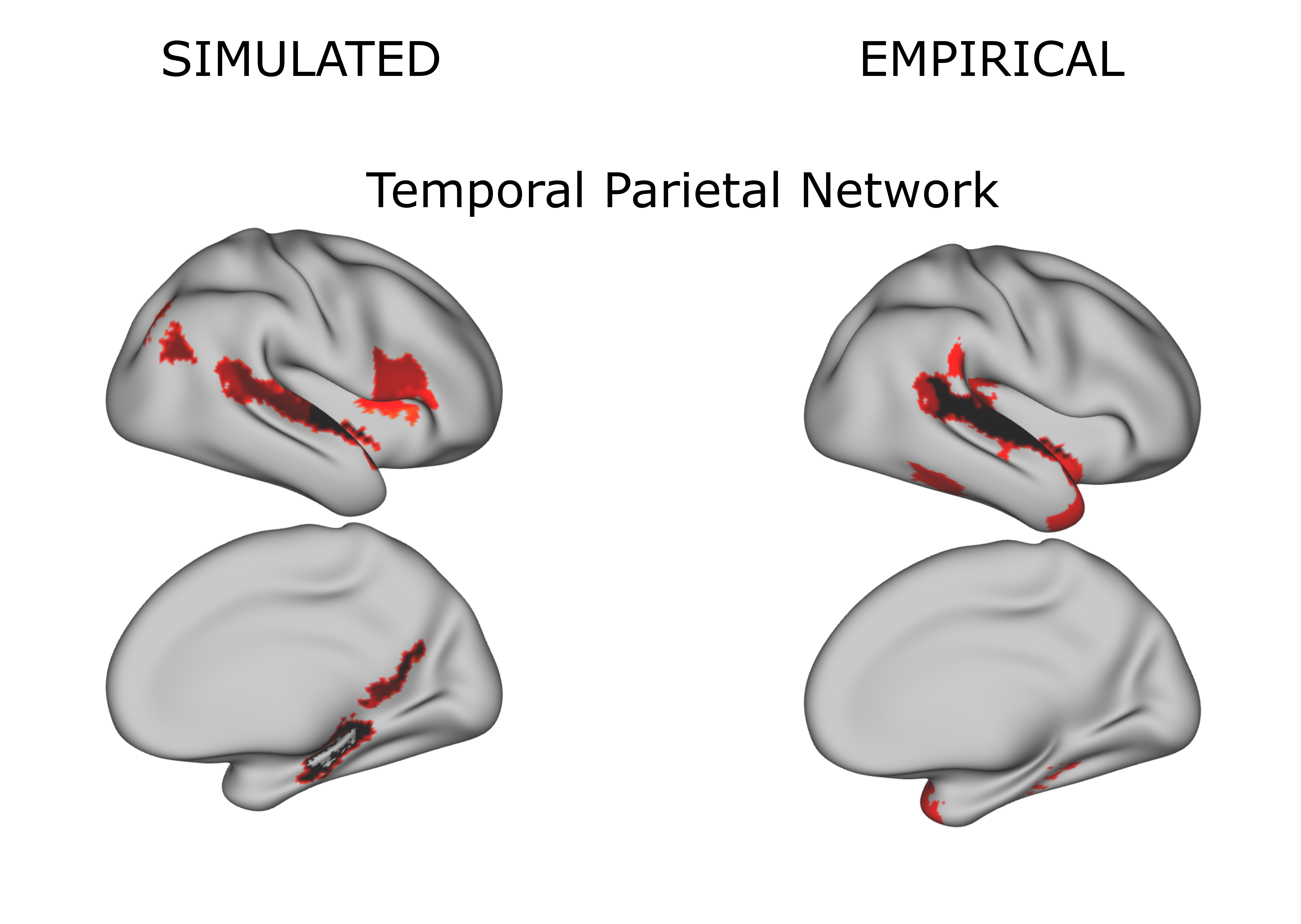
Methods
- Dynamic modelling (Dynamic Mean Field model, Hopf model, etc.)
- Connectivity analyses (structural connectivity, static functional connectivity, dynamic functional connectivity, effective connectivity)
- Structural and functional MRI preprocessing (and creation of preprocessing pipelines)
- Resting state and task-based fMRI analyses
- Neurophysiology (TMS, EMG, EEG)
- Medical informatics
- Open science